simpeg.directives.BetaEstimate_ByEig#
- class simpeg.directives.BetaEstimate_ByEig(beta0_ratio=1.0, n_pw_iter=4, seed=None, **kwargs)[source]#
Bases:
BaseBetaEstimator
Estimate initial trade-off parameter (beta) by power iteration.
The initial trade-off parameter (beta) is estimated by scaling the ratio between the largest eigenvalue in the second derivative of the data misfit and the model objective function. The largest eigenvalues are estimated using the power iteration method; see
simpeg.utils.eigenvalue_by_power_iteration()
. The estimated trade-off parameter is used to update the beta property in the associatedsimpeg.inverse_problem.BaseInvProblem
object prior to running the inversion. Note that a separate directive is used for updating the trade-off parameter at successive beta iterations; seeBetaSchedule
.- Parameters:
- beta0_ratio: float
Desired ratio between data misfit and model objective function at initial beta iteration.
- n_pw_iter
int
Number of power iterations used to estimate largest eigenvalues.
- seed
None
orRandomSeed
,optional
Random seed used for random sampling. It can either be an int, a predefined Numpy random number generator, or any valid input to
numpy.random.default_rng
.
Notes
Let
represent the data misfit, represent the model objective function and represent the starting model. The first model update is obtained by minimizing the a global objective function of the form:where
represents the initial trade-off parameter (beta). Let define the desired ratio between the data misfit and model objective functions at the initial beta iteration (defined by the ‘beta0_ratio’ input argument). Using the power iteration approach, our initial trade-off parameter is given by:where
as the largest eigenvalue of the Hessian of the data misfit, and as the largest eigenvalue of the Hessian of the model objective function. For each Hessian, the largest eigenvalue is computed using power iteration. The input parameter ‘n_pw_iter’ sets the number of power iterations used in the estimate.For a description of the power iteration approach for estimating the larges eigenvalue, see
simpeg.utils.eigenvalue_by_power_iteration()
.Attributes
The estimated ratio is multiplied by this to obtain beta.
verbose.debug has been deprecated.
Data misfit associated with the directive.
Inverse problem associated with the directive.
Inversion object associated with the directive.
Number of power iterations for estimating largest eigenvalues.
Optimization algorithm associated with the directive.
Regularization associated with the directive.
Random seed to initialize with.
Return simulation for all data misfits.
Return survey for all data misfits
Whether or not to print debugging information.
Methods
endIter
()Update inversion parameter(s) according to directive at end of iteration.
finish
()Update inversion parameter(s) according to directive at end of inversion.
Initialize inversion parameter(s) according to directive.
validate
(directive_list)Validate directive.
Galleries and Tutorials using simpeg.directives.BetaEstimate_ByEig
#
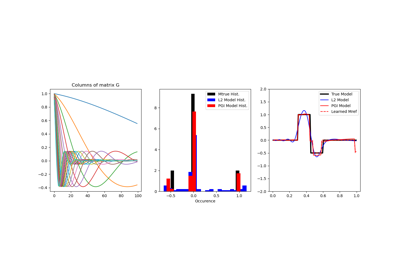
Petrophysically guided inversion (PGI): Linear example
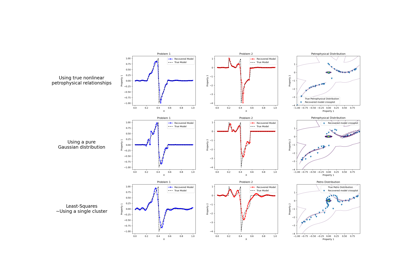
Petrophysically guided inversion: Joint linear example with nonlinear relationships
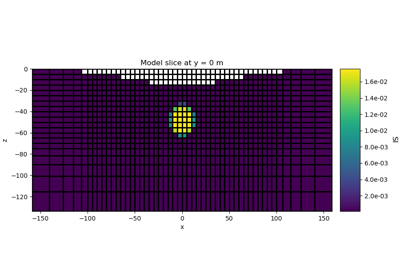
Sparse Norm Inversion for Total Magnetic Intensity Data on a Tensor Mesh

Joint PGI of Gravity + Magnetic on an Octree mesh using full petrophysical information

Joint PGI of Gravity + Magnetic on an Octree mesh without petrophysical information
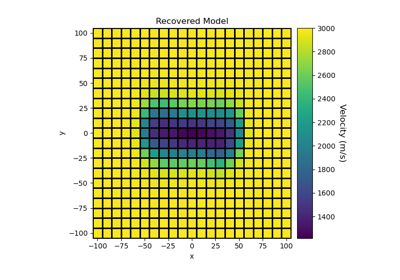
Sparse Norm Inversion of 2D Seismic Tomography Data
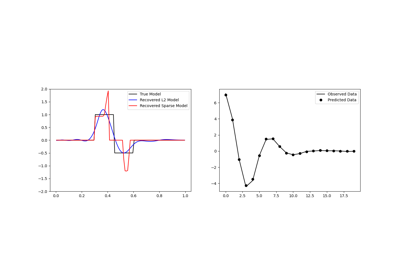
Sparse Inversion with Iteratively Re-Weighted Least-Squares
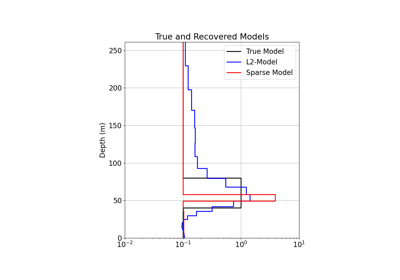
1D Inversion of Time-Domain Data for a Single Sounding
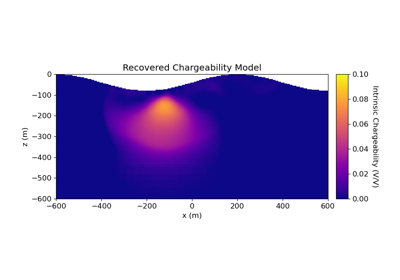
2.5D DC Resistivity and IP Least-Squares Inversion