simpeg.directives.UpdateSensitivityWeights#
- class simpeg.directives.UpdateSensitivityWeights(every_iteration=False, threshold_value=1e-12, threshold_method='amplitude', normalization_method='maximum', **kwargs)[source]#
Bases:
InversionDirective
Sensitivity weighting for linear and non-linear least-squares inverse problems.
This directive computes the root-mean squared sensitivities for the forward simulation(s) attached to the inverse problem, then truncates and scales the result to create cell weights which are applied in the regularization. The underlying theory is provided below in the Notes section.
This directive requires that the map for the regularization function is either class:simpeg.maps.Wires or class:simpeg.maps.Identity. In other words, the sensitivity weighting cannot be applied for parametric inversion. In addition, the simulation(s) connected to the inverse problem must have a
getJ
orgetJtJdiag
method.This directive’s place in the
DirectivesList
must be before any directives which update the preconditioner for the inverse problem (i.e.UpdatePreconditioner
), and must be before any directives that estimate the starting trade-off parameter (i.e.EstimateBeta_ByEig
andEstimateBetaMaxDerivative
).- Parameters:
- every_iterationbool
When
True
, update sensitivity weighting at every model update; non-linear problems. WhenFalse
, create sensitivity weights for starting model only; linear problems.- threshold
float
Threshold value for smallest weighting value.
- threshold_method{‘amplitude’, ‘global’, ‘percentile’}
Threshold method for how threshold_value is applied:
- amplitude:
the smallest root-mean squared sensitivity is a fractional percent of the largest value; must be between 0 and 1.
- global:
threshold_value is added to the cell weights prior to normalization; must be greater than 0.
- percentile:
the smallest root-mean squared sensitivity is set using percentile threshold; must be between 0 and 100.
- normalization_method{‘maximum’, ‘min_value’,
None
} Normalization method applied to sensitivity weights.
Options are:
- maximum:
sensitivity weights are normalized by the largest value such that the largest weight is equal to 1.
- minimum:
sensitivity weights are normalized by the smallest value, after thresholding, such that the smallest weights are equal to 1.
None
:normalization is not applied.
Attributes
verbose.debug has been deprecated.
Data misfit associated with the directive.
every_iteration.everyIter has been deprecated.
Update sensitivity weights when model is updated.
Inverse problem associated with the directive.
Inversion object associated with the directive.
normalization_method.normalization has been deprecated.
Normalization method applied to sensitivity weights.
Optimization algorithm associated with the directive.
Regularization associated with the directive.
Return simulation for all data misfits.
Return survey for all data misfits
threshold_value.threshold has been deprecated.
Threshold method for how threshold_value is applied:
Threshold value used to set minimum weighting value.
Whether or not to print debugging information.
Methods
endIter
()Execute end of iteration.
finish
()Update inversion parameter(s) according to directive at end of inversion.
Compute sensitivity weights upon starting the inversion.
update
()Update sensitivity weights
validate
(directiveList)Validate directive against directives list.
Notes
Let
represent the Jacobian. To create sensitivity weights, root-mean squared (RMS) sensitivities are computed by summing the squares of the rows of the Jacobian:The dynamic range of RMS sensitivities can span many orders of magnitude. When computing sensitivity weights, thresholding is generally applied to set a minimum value.
Thresholding:
If global thresholding is applied, we add a constant
to the RMS sensitivities:In the case of percentile thresholding, we let
represent a given percentile. Thresholding to set a minimum value is applied as follows:If absolute thresholding is applied, we define
as a fractional percent. In this case, thresholding is applied as follows:
Galleries and Tutorials using simpeg.directives.UpdateSensitivityWeights
#
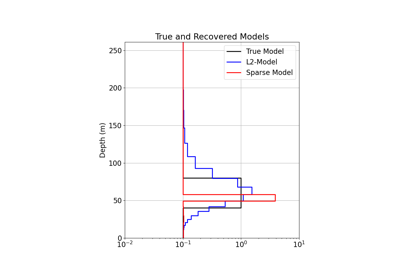
1D Inversion of Time-Domain Data for a Single Sounding
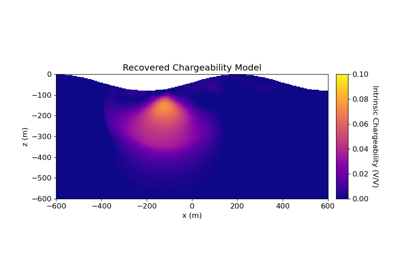
2.5D DC Resistivity and IP Least-Squares Inversion
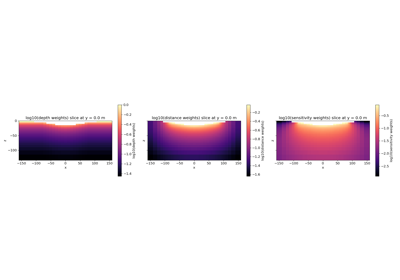
Compare weighting strategy with Inversion of surface Gravity Anomaly Data
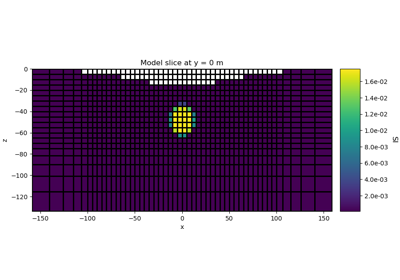
Sparse Norm Inversion for Total Magnetic Intensity Data on a Tensor Mesh
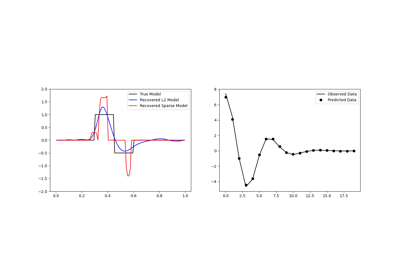
Sparse Inversion with Iteratively Re-Weighted Least-Squares
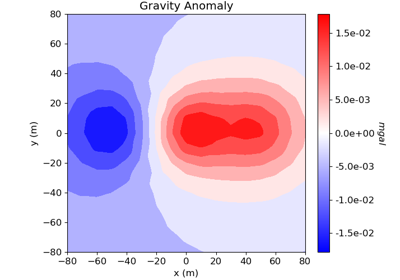
Cross-gradient Joint Inversion of Gravity and Magnetic Anomaly Data