SimPEG.utils.WeightedGaussianMixture#
- class SimPEG.utils.WeightedGaussianMixture(n_components, mesh, actv=None, covariance_type='full', init_params='kmeans', max_iter=100, means_init=None, n_init=10, precisions_init=None, random_state=None, reg_covar=1e-06, tol=0.001, verbose=0, verbose_interval=10, warm_start=False, weights_init=None)[source]#
Bases:
sklearn.mixture._gaussian_mixture.GaussianMixture
Weighted Gaussian mixture class
This class upon the GaussianMixture class from Scikit-Learn. Two main modifications:
1. Each sample/observation is given a weight, the volume of the corresponding discretize.BaseMesh cell, when fitting the Gaussian Mixture Model (GMM). More volume gives more importance, ensuing a mesh-free evaluation of the clusters of the geophysical model.
2. When set manually, the proportions can be set either globally (normal behavior) or cell-by-cell (improvements)
Disclaimer: this class built upon the GaussianMixture class from Scikit-Learn. New functionalitie are added, as well as modifications to existing functions, to serve the purposes pursued within SimPEG. This use is allowed by the Scikit-Learn licensing (BSD-3-Clause License) and we are grateful for their contributions to the open-source community.
Addtional parameters to provide, compared to sklearn.mixture.gaussian_mixture:
- Parameters
- n_components
int
Number of components
- mesh
discretize.BaseMesh
TensorMesh
orQuadTree
or Octree) mesh: the volume of the cells give each sample/observations its weight in the fitting proces- actv
numpy.ndarry
,optional
Active indexes
- n_components
Methods
Compute the precisions matrices and their Cholesky decomposition.
Compute and set the precisions matrices and their Cholesky decomposition.
order_clusters_GM_weight
([outputindex])Order clusters by decreasing weights
plot_pdf
([ax, flag2d, x_component, ...])Utils to plot the marginal PDFs of a GMM, either in 1D or 2D (1 or 2 physical properties at the time).
score
(X[, y])Compute the per-sample average log-likelihood
score_samples_with_sensW
(X, sensW)Compute the weighted log probabilities for each sample.
Galleries and Tutorials using SimPEG.utils.WeightedGaussianMixture
#
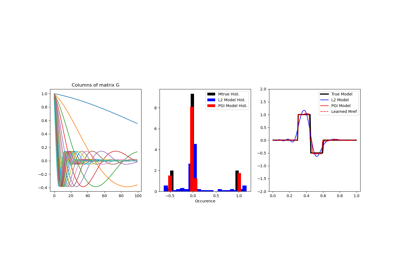
Petrophysically guided inversion (PGI): Linear example
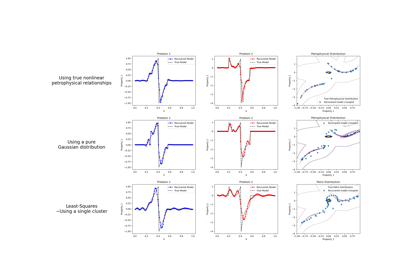
Petrophysically guided inversion: Joint linear example with nonlinear relationships

Joint PGI of Gravity + Magnetic on an Octree mesh using full petrophysical information

Joint PGI of Gravity + Magnetic on an Octree mesh without petrophysical information